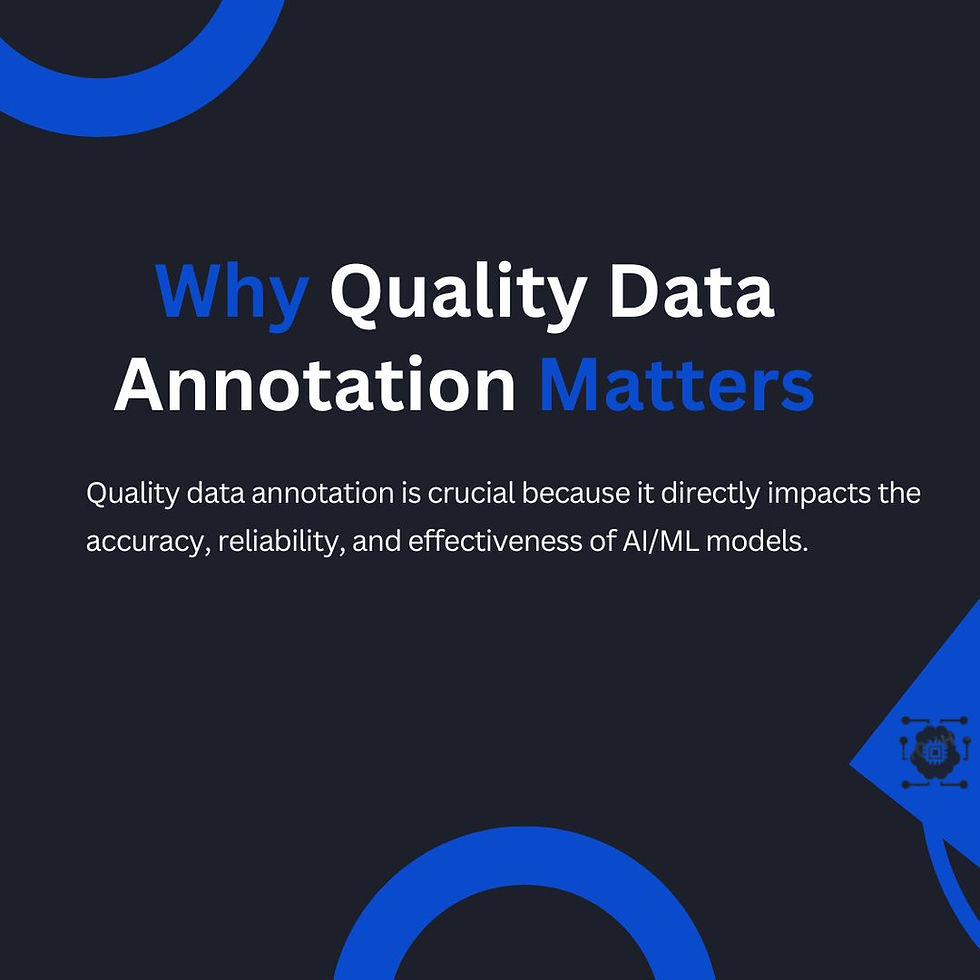
In the rapidly evolving field of Artificial Intelligence (AI) and Machine Learning (ML), the accuracy and effectiveness of models largely depend on the quality of the data they are trained on. Data annotation, the process of labeling data to teach AI/ML models how to recognize patterns and make decisions, plays a crucial role in this process. High-quality data annotation is the foundation of successful AI/ML applications, making it a critical area for businesses and researchers alike. In this blog, we'll explore the importance of quality in data annotation, key considerations to keep in mind, and some best practices to ensure your annotations are up to standard.
Why Quality Data Annotation Matters.
Imagine you're developing an AI model to detect and classify different types of vehicles in images. To do this effectively, the model needs to be trained on a large dataset of images where vehicles are accurately labeled. If the annotations are inconsistent (e.g., some cars are labeled as trucks or some vehicles are missed entirely), the model will struggle to make accurate predictions. This is why quality in data annotation is so crucial.
Poorly annotated data can lead to a range of issues, including:
Inaccurate Model Predictions: The AI/ML model may learn incorrect patterns or fail to recognize important features.
Increased Costs and Time: Low-quality annotations can require rework, leading to increased costs and delays in project timelines.
Reduced Trust: Inconsistent or inaccurate models can erode trust in AI systems, especially in critical applications like healthcare or autonomous driving.
Key Quality Considerations in Data Annotation
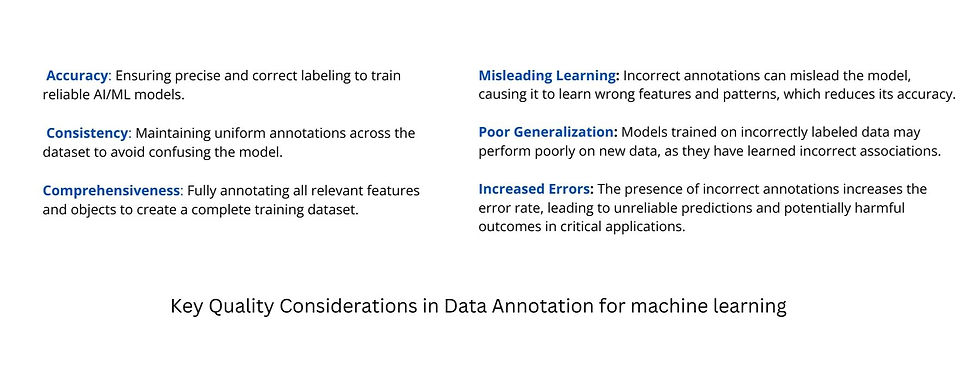
To ensure your AI/ML models perform optimally, consider the following quality queries during the data annotation process:
Are the Annotations Consistent and Accurate?
Consistency is key in data annotation. For example, if you're annotating images of cats and dogs, ensure that all instances of cats are labeled as "cat" and all dogs as "dog." Inconsistent labeling can confuse the model and reduce its effectiveness. Accuracy is equally important; even small errors in labeling can lead to significant issues in model performance.
Have All Relevant Objects and Features Been Annotated?
Comprehensive annotation ensures that no critical elements are missed. For instance, if you're annotating traffic scenes, it's important to label all relevant objects such as cars, pedestrians, traffic lights, and road signs. Missing annotations can lead to incomplete training data, which affects the model's ability to make accurate predictions.
Is There a Robust Quality Control Process in Place?
Quality control mechanisms, such as having multiple annotators review the same data or using automated tools to check for errors, are essential for maintaining high annotation standards. For example, a review process could catch that a specific type of vehicle was consistently mislabeled, allowing for corrections before the data is used to train the model.
Best Practices for Ensuring High-Quality Data Annotation
To achieve the best results in your AI/ML projects, follow these best practices for data annotation:
Clear Annotation Guidelines: Provide detailed and unambiguous guidelines to annotators to ensure consistency. For example, if you're working on facial recognition, specify how to label features like eyes, nose, and mouth.
Balanced Datasets: Ensure that your dataset includes a balanced representation of different classes or categories. For instance, in a dataset of animal images, have an equal number of cat and dog images to avoid bias.
Edge Case Handling: Pay special attention to unusual or edge cases, such as blurry images or partial objects. Properly annotating these can significantly improve model robustness.
Continuous Quality Checks: Implement ongoing quality checks throughout the annotation process. This can include spot checks, inter-annotator agreement analysis, and regular feedback sessions with annotators.
Real-World Example: Data Annotation in Autonomous Driving
Consider an AI/ML project focused on autonomous driving. Here, data annotation involves labeling objects such as vehicles, pedestrians, lane markings, and traffic signals in video frames. Consistency and accuracy are paramount because any mistake could lead to life-threatening errors in real-world applications. For instance, if a pedestrian is not correctly labeled in a training dataset, the autonomous vehicle might fail to detect pedestrians in actual driving scenarios, leading to potentially dangerous situations.
By ensuring high-quality data annotation, the model can be trained to recognize and respond to various driving conditions accurately, making autonomous vehicles safer and more reliable.
Conclusion
In the world of AI and ML, quality data annotation is not just a step in the process—it's the cornerstone of success. Whether you're working on image recognition, natural language processing, or any other AI/ML application, ensuring that your data is accurately and consistently annotated will lead to better model performance and more reliable outcomes.
If you're looking to elevate your AI/ML projects with high-quality data annotation, Generative Insights offers expert annotation solutions tailored to your specific needs. Explore our blog to learn more about the latest trends, technologies, and best practices in AI/ML data annotation.
This blog is optimized for SEO with keywords like "data annotation," "AI/ML," "quality data annotation," and "best practices in data annotation." It is designed to be both informative and engaging, helping to attract readers interested in AI and machine learning.
Comments