What is Data Annotation? Its Types, Role, Challenges, and Solutions - AI Project on hand experiences
The field of data annotation is vast, and at the moment, the AI market is concentrated on feature extraction from billions of photos, where data annotation is essential to a subset of machine learning.
Let's begin by concentrating on how HITL uses data annotation to assist machines in learning complex environments.
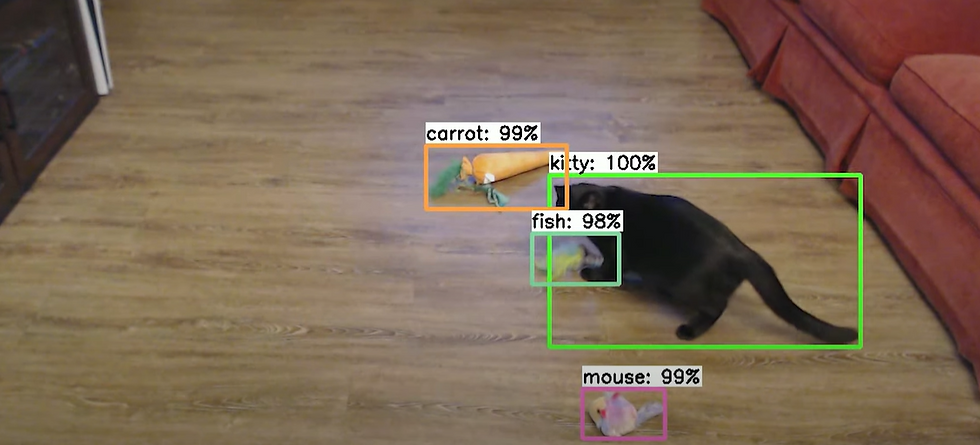
In today’s data-driven world, businesses and organizations are increasingly relying on machine learning (ML) and artificial intelligence (AI) to gain insights and make informed decisions. However, for these systems to perform accurately, they require large volumes of high-quality data that is properly annotated. This is where data annotation comes into play.
What is Data Annotation?
Data annotation is the process of labeling or adding metadata to raw data to make it understandable by machines. It involves adding meaning to data, which could include labeling images, tagging text, identifying objects in videos, or transcribing audio into text. The primary goal of data annotation is to create structured data that can be used to train machine learning models, ultimately improving their accuracy and performance.
Why is Data Annotation Important?
The importance of data annotation cannot be overstated in the context of AI and machine learning. Properly annotated data is the backbone of any successful ML model. Without it, machines would struggle to understand the context and nuances of the data they are processing, leading to inaccurate predictions and unreliable outcomes. High-quality data annotation enables AI models to learn from the data more effectively, resulting in better performance and more reliable outputs.
Types of Data Annotation
Data annotation encompasses various types, each catering to different needs and applications:
1. Image Annotation : This involves labeling images with metadata such as identifying objects, drawing bounding boxes, or categorizing images into different classes. Image annotation is crucial for computer vision tasks like object detection, facial recognition, and image segmentation.
2. Text Annotation: Text annotation involves tagging and labeling textual data, which can include annotating entities, sentiment analysis, and part-of-speech tagging. This type is vital for natural language processing (NLP) tasks such as chatbots, sentiment analysis, and machine translation.
3. Audio Annotation: In this type, audio files are transcribed into text or labeled for specific sounds, speech patterns, or intonations. It is essential for speech recognition systems, voice assistants, and audio-based sentiment analysis.
4. Video Annotation: Video annotation includes labeling objects, actions, or events within video frames. It is particularly important for applications in autonomous driving, surveillance, and action recognition in videos.
5. 3D Point Cloud Annotation: This involves labeling 3D data points collected by LiDAR scanners. It’s crucial for applications like autonomous driving, where understanding the 3D environment is essential.
The Role of Data Annotation in AI
Data annotation plays a pivotal role in the development and deployment of AI models. It ensures that the data fed into machine learning algorithms is clean, structured, and relevant, enabling the models to learn from it effectively. Proper data annotation improves the accuracy of AI models, making them more reliable in real-world applications.
Challenges in Data Annotation
Despite its importance, data annotation comes with several challenges:
1. Time-Consuming Process: Data annotation is often a manual and labor-intensive process, requiring significant time and effort, especially when dealing with large datasets.
2. High Cost: The need for human annotators and the sheer volume of data required can make data annotation an expensive endeavor.
3. Inconsistency: Inconsistent labeling by different annotators can lead to poor data quality, which in turn affects the performance of the AI models.
4. Scalability: As the volume of data grows, scaling the annotation process becomes increasingly difficult without compromising on quality.
Solutions to Data Annotation Challenges
To overcome these challenges, organizations can adopt several strategies:
1. Automation: Leveraging automated tools and AI-based platforms can speed up the annotation process and reduce costs.
2. Quality Control: Implementing rigorous quality control measures, such as cross-checking annotations and using consensus algorithms, can ensure consistency and accuracy in the data.
3. Outsourcing: Outsourcing data annotation to specialized firms can help scale the process without the need for significant in-house resources.
4. Active Learning: Integrating active learning techniques allows models to prioritize the annotation of data that is most informative, reducing the amount of data that needs to be labeled manually.
Conclusion
Data annotation is a critical component in the AI and machine learning pipeline. It transforms raw data into a format that machines can understand, making it possible to train accurate and reliable AI models. While the process comes with its own set of challenges, adopting the right strategies can help organizations overcome these hurdles and unlock the full potential of their AI initiatives.
For businesses looking to stay ahead in the AI race, investing in high-quality data annotation services is not just an option—it’s a necessity.
Comments